Using Polar2grid and Direct Broadcast files to create imagery
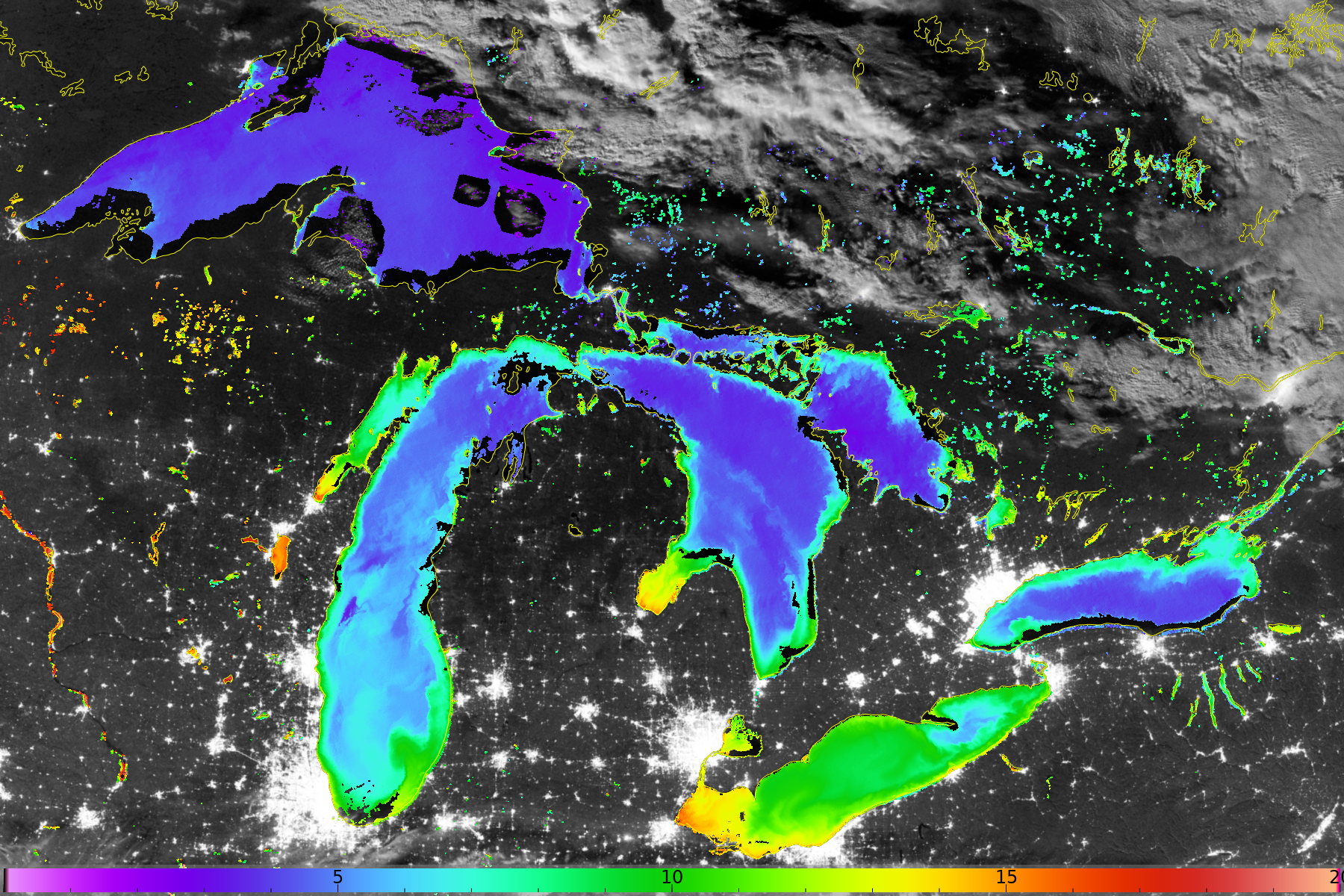
It was another clear night over the Great Lakes on 12 May 2025 and NOAA-20 had a descending orbit over western lower Michigan around 0740 UTC that allowed a view of all five Great Lakes. CSPP software running at CIMSS produces both SDR (Sensor Data Record) and EDR (Environmental Data Record) files that can be... Read More